Accessing Institutional S3 Object Storage
We published the following unclassified unlimited release (UUR) technical report advocating for S3 storage use.
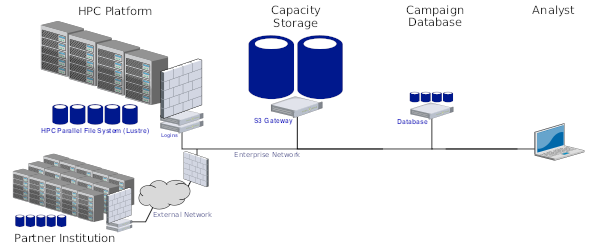
Abstract
Recent efforts at Sandia such as DataSEA are creating search engines that enable analysts to query the institution's massive archive of simulation and experiment data. The benefit of this work is that analysts will be able to retrieve all historical information about a system component that the institution has amassed over the years and make better-informed decisions in current work. As DataSEA gains momentum, it faces multiple technical challenges relating to capacity storage. From a raw capacity perspective, data producers will rapidly overwhelm the system with massive amounts of data. From an accessibility perspective, analysts will expect to be able to retrieve any portion of the bulk data, from any system on the enterprise network. Sandia's Institutional Computing is mitigating storage problems at the enterprise level by procuring new capacity storage systems that can be accessed from anywhere on the enterprise network. These systems use the simple storage service, or S3, API for data transfers. While S3 uses objects instead of files, users can access it from their desktops or Sandia's high-performance computing (HPC) platforms. S3 is particularly well suited for bulk storage in DataSEA, as datasets can be decomposed into object that can be referenced and retrieved individually, as needed by an analyst.
In this report we describe our experiences working with S3 storage and provide information about how developers can leverage Sandia's current systems. We present performance results from two sets of experiments. First, we measure S3 throughput when exchanging data between four different HPC platforms and two different enterprise S3 storage systems on the Sandia Restricted Network (SRN). Second, we measure the performance of S3 when communicating with a custom-built Ceph storage system that was constructed from HPC components. Overall, while S3 storage is significantly slower than traditional HPC storage, it provides significant accessibility benefits that will be valuable for archiving and exploiting historical data. There are multiple opportunities that arise from this work, including enhancing DataSEA to leverage S3 for bulk storage and adding native S3 support to Sandia's IOSS library.
Publication
- SAND Report Todd Kordenbrock, Gary Templet, Craig Ulmer, and Patrick Widener, "Viability of S3 Object Storage for the ASC Program at Sandia". SAND2022-1538, October 2022.